The speakers will provide pre-recorded lectures. Free slots allow you to watch those lectures before the Q&A sessions.
CET | Monday 17/01 |
Tuesday 18/01 | Wednesday 19/01 | Thursday 20/01 |
Friday 21/01 |
---|---|---|---|---|---|
8-9 | |||||
9-10 | |||||
10-11 | |||||
11-12 | Gather Town Social Session 2 | Zoom Q&A Djamé Seddah |
|||
12-13 | |||||
13-14 | Gather town Poster Session 1 |
Zoom Q&A Iryna Gurevych and Jonas Pfeiffer |
|||
14-15 | Zoom Q&A Colin Raffel |
Slack Lab Session Adapters |
|||
15-16 | Gather TownSocial Session 1 | Zoom Q&A Kyunghyun Cho |
|||
16-17 | Slack Lab Session Neural NMT |
Gather town Poster Session 2 |
|||
17-18 | Zoom Q&A Graham Neubig |
Zoom Q&A Yejin Choi |
|||
18-19 | Zoom Q&A Mona Diab |
Poster Sessions
Session 1
-
A 1 Daryna Dementieva
Methods of Detoxification of Texts for the Russian Language[Abstract]
We introduce the first study of the automatic detoxification of Russian texts to combat offensive language. This kind of textual style transfer can be used for processing toxic content on social media or for eliminating toxicity in automatically generated texts. While much work has been done for the English language in this field, there are no works on detoxification for the Russian language. We suggest two types of models—an approach based on BERT architecture that performs local corrections and a supervised approach based on a pretrained GPT-2 language model. We compare these methods with several baselines. In addition, we provide the training datasets and describe the evaluation setup and metrics for automatic and manual evaluation. The results show that the tested approaches can be successfully used for detoxification, although there is room for improvement. -
A 2 Zae Myung Kim
Visualizing Cross-Lingual Discourse Relations in Multilingual TED Corpora[Abstract]
This paper presents an interactive data dashboard that provides users with an overview of the preservation of discourse relations among 28 language pairs. We display a graph network depicting the cross-lingual discourse relations between a pair of languages for multilingual TED talks and provide a search function to look for sentences with specific keywords or relation types, facilitating ease of analysis on the cross-lingual discourse relations. -
A 3 Ashutosh Kumar
Inducing diversity and syntactic control in paraphrase generation[Abstract]
Paraphrasing is the task of rephrasing a given text in multiple ways such that the semantics of the generated sentences remain unaltered. We present two methods for generating such paraphrases with one method focusing on what to generate, while the other focusing on how to generate. Specifically, we use submodular optimization to address the former and syntactic signals for the latter. -
A 4 David Dale
Teaching a general conversation model to be less toxic[Abstract]
Modern language models are apt at generating natural and fluent dialogue responses. However, after training on large web corpora, the models absorb the toxicity present therein and reproduce it uncontrollably. However, using a toxicity classifier and RL to reward the model during training for generating non-toxic responses to provocative utterances largely mitigates this problem. We train a small conversational model for English and Russian and show that it can be guided towards safer talk. -
A 5 Richard Plant
CAPE: Context-Aware Private Embeddings for Private Language Learning[Abstract]
Neural language models have contributed to state-of-the-art results, however obtaining embeddings using these models risks revealing personal information that may lead to privacy leaks. We propose Context-Aware Private Embeddings (CAPE), a novel approach which combines differential privacy and adversarial learning to preserve privacy during training of embeddings. CAPE applies calibrated noise through differential privacy to maintain the privacy of text representations by preserving the encoded semantic links while obscuring sensitive information. CAPE employs an adversarial training regime that obscures identified private variables. Experimental results demonstrate that our proposed approach is more effective in reducing private information leakage than either single intervention, with approximately a 3% reduction in attacker performance compared to the best-performing current method. -
A 6 Mitja Nikolaus
Modeling the Interaction Between Perception-Based and Production-Based Learning in Children’s Early Acquisition of Semantic Know[Abstract]
Children learn the meaning of words and sentences in their native language at an impressive speed and from highly ambiguous input. To account for this learning, previous computational modeling has focused mainly on the study of perception-based mechanisms like cross-situational learning. However, children do not learn only by exposure to the input. As soon as they start to talk, they practice their knowledge in social interactions and they receive feedback from their caregivers. In this work, we propose a model integrating both perception- and production-based learning using artificial neural networks which we train on a large corpus of crowd-sourced images with corresponding descriptions. We found that production-based learning improves performance above and beyond perception-based learning across a wide range of semantic tasks including both word- and sentence-level semantics. In addition, we documented a synergy between these two mechanisms, where their alternation allows the model to converge on more balanced semantic knowledge. The broader impact of this work is to highlight the importance of modeling language learning in the context of social interactions where children are not only understood as passively absorbing the input, but also as actively participating in the construction of their linguistic knowledge. -
A 8 Sofiya Kobylyanskaya
AAnalysis of spoken language variation for second language (L2) acquisition in multimodal settings[Abstract]
The linguistic study of the spoken langage variation is increasingly considered in multimodal settings. Such new frameworks may include expertise from both computer, human and social sciences. The project «LeCycl» is part of this scientific trend and focuses on the acquisition of types of knowledge through various multimodal measures. My work is thus focusing on second langage (L2) acquisition via the study of the linguistic variation combined with eye-tracking measures. Its goal is to underpin the analysis of the variation in L2 speech using additional information about reading strategies, text comprehension by the participants and their level of L2 mastering. The poster describes the experimental protocol and preliminary linguistic analysis of acoustic variation in a corpus of native Japanese speakers reading English (L2) sentences. -
A 9 Khrystyna Skopyk
Personalizing Large Language Models[Abstract]
Much work on personalization of large language models is done in the area of dialog, summary or review generation. Previously, many approaches used sequence-to-sequence architectures with different tricks and persona-related meta-data, which is an intricate work. A few recent papers, suggest text personalization methods using only previously written texts as input and transformer-like architecture along with fine-tuning. This work will be focused on personalization as an autocomplete task, using such methods as fine-tuning, few-shot learning, and meta-learning. -
A 10 Saliha Muradoglu
Testing the linguistics of transformer generalizations[Abstract]
Neural sequence-to-sequence models have been very successful at tasks in phonology and morphology that seemingly require a capacity for intricate linguistic generalizations. In this paper we perform a detailed breakdown of the power of such models to capture various phonological generalizations and to benefit from exposure to one phonological rule to infer the behavior of another similar rule. We present two types of experiments, one which establishes the efficacy of the transformer model on 29 different processes. The second experiment type follows a priming and held-out case split where our model is exposed to two (or more) phenomena; one which is used as a primer to make the model aware of a linguistic category (e.g. voiceless stops) and a second one which contains a rule with a withheld case that the model is expected to infer (e.g. word-final devoicing with a missing training example such as b>p). -
A 11 Lily Wadoux
Voice cloning for pathological speech: impact of the linguistic content[Abstract]
In the domain of speech synthesis, voice cloning is the process of producing speech matching a target speaker voice, given textual input. Voice cloning systems are based on neural Text-to-Speech approaches, trained on large multi-speaker corpora. Coupled to a speaker encoder, they can achieve high-quality speech with only few data from the target speaker. A target speaker with impaired speech may only produce speech with specific or limited linguistic content. To our knowledge, the impact of such constraints has yet to be studied. This poster presents the work in progress of an ongoing PhD on the matter, with its first results and specifications about the models and datasets used. -
A 12 Mut Altın Lütfiye Seda
Automatic Detection of Sexism in Social Media[Abstract]
Sexism is defined as discriminative actions or attitudes against people based on their gender. Social media provides a space for unregulated gender-based cyber-hate again certain groups. Due to the challenges of moderation, automatic detection of sexist language in social media gains a lot of traction. We participated to EXIST shared task (Iberlef 2021) and approached the problem with a transformers-based system applying a multi-lingual BERT model based on the shared task dataset that contains Tweets in English and Spanish. And the classification was done first: binary as sexist, not-sexist and second: a multi-class classification to be able to spot the type of sexism and promising results were obtained. Detailed published recently within the scope of IberLef. (http://ceur-ws.org/Vol-2943/exist_paper8.pdf) -
A 13 Ali Safaya
Disentangled Entity State Modeling via Co-reference Informed Memory Pre-training[Abstract]
Entity state modeling is a challenging task in the field of natural language processing. Current training objectives of language models do not provide us with the ability to model the changes of entity states throughout passages explicitly. In our ongoing work, we propose an entity state modeling approach based on co-reference informed memory pre-training. The proposed model is a combination of a pre-trained language model, a neural disentangled entity memory of dynamic size, and a co-reference-aware memory updating module. We believe the proposed model can effectively capture the entity state dynamics in a text and learn to encode disentangled entity representations thus improve the performance of entity state modeling. -
A 14 Sergey Pletenev
The autoregressive structures out of non-autoregressive space[Abstract]
Since the popularization of the Transformer as a general purpose feature encoder for NLP, many studies have attempted to decode linguistic structure from its novel multi-head attention mechanism. However, much of such work focused almost exclusively on autoregressive style of decoding. In this study, we present decoding experiments on Non-autoregessive models in order to test the generalizability of the claim that dependency syntax is reflected in attention patterns -
A 15 Lucía Ormaechea Grijalba
Integrating Grammar-Based Language Models into Domain-Specific ASR Systems[Abstract]
Language Models (LMs) represent a crucial component in the architecture of Automatic Speech Recognition (ASR) systems. Current trends in this area point to the creation of high-performing and increasingly robust systems through the exploitation of large amounts of data. Even though the use of corpus-based models proves to be a dominant strategy for language modelling, it may not be the most suitable approach in some of today’s ASR applications. This is especially evident in domains where there is a strong interest in controlling the hypotheses generated by the system and producing only reliable outputs. Providing a deliberately constrained transcription can be more effectively achieved using a formal approach, and thus with the use of grammars, which ultimately contribute to better capturing the inherent structures of the target language. For these reasons, we present a tool that allows to efficiently integrate regular grammars as LMs in Kaldi, a widely used toolkit for speech recognition research. To the best of our knowledge, there is currently no existing tool that performs this task. We thus make it freely available along with some demo examples and crowdsourced evaluation corpora so that it can be used by researchers or developers in their own experiments and applications. -
A 16 Oskar Van der Wal
The Birth of Bias in Large Language Models[Abstract]
There is a growing concern about the use of Large Language Models (LLMs), as they have been shown to exhibit undesirable biases, but its mitigation remains challenging. We believe that it is crucial to study how LLMs come to be biased in the first place, and what role the training data, architecture, and downstream application play at various phases in the life-cycle of an NLP model. In this paper, we work towards a better understanding of the origins of bias in LLMs by investigating the learning dynamics of gender bias, and our findings suggest that the bias representation is dynamic in multiple ways. Moreover, we shed light on the relationship between the intrinsic parameters and the extrinsic biased behaviour. -
A 17 Nicolas David
Enriching resource-poor languages through Transformer-based NLP models[Abstract]
In the field of Natural Language Processing (NLP), resource-poor languages represent a challenging topic and much interest towards their enrichment has been demonstrated, especially through the ever-growing research and development of NLP models to accomplish various NLP tasks. With the advent of neural network architectures, this enrichment process has undergone significant progress and newer architectures, like Transformer-based NLP models, tend to further accelerate this process, while outperforming existing ones. In this context, this research work, as part of an ongoing PhD Thesis, aims at harnessing these models in the view of modelling the linguistic resources of a French-based creole language: the Mauritian Creole. Supported by a Treebank, this work in progress hence focuses on a specific NLP task: dependency parsing. -
A 18 Tristan Luiggi
Contextual Named Entity Recognition[Abstract]
Named Entity Recognition (NER) is a well-studied field of NLP consisting in detecting entities (places, persons, locations…) within a text. Recent advances in deep learning have shown high performances via new models such as LSTM-CRF or Transformers. All the solutions still present limitations, as an entity is usually classified into generic classes that do not heavily rely on the context of its utilization. What if an entity is used in a different context and therefore is not always labeled in the same way depending on the context? Our work focuses on such a situation which we call Contextualized Named Entity Recognition. We propose the formulation of a novel task along with two dataset benchmarks: detecting winning/losing players in basketball match summaries and detecting actors and their credit order with movies synopsis. We evaluate baseline models using common metrics such as precision/recall/F1 on transformer-based architecture. Finally, we present experiments reflecting issues and research axes related to the novel task.
Session 2
-
B 1 Attila Nagy
Syntax-aware data augmentation for neural machine translation[Abstract]
Data augmentation methods in machine translation in most cases alter only the source-side sentences and leave the sentence of the target language untouched. In case of blanking or replacement of arbitrary words, the correctness of translation can easily be harmed, resulting in incorrect translations. I have been working on a method for creating a syntax-aware data augmentation method for NMT: swapping specific subtrees of the dependency trees for the source and target sentences simultaneously. -
B 2 Everlyn Chimoto
Neural Machine Translation for Low-Resource Languages[Abstract]
Neural Machine Translation has achieved state-of-the-art performance in the past few years with numerous languages. Despite this performance, African languages have not benefited adequately from Neural Machine Translation. This study ameliorates the state of Neural Machine Translation for African languages by applying the transformer model architecture to 3 African languages. Namely, Kinyarwanda, Luganda and Luhya. First, baseline models were developed. These models achieved a BLEU score of 38.67, 37.28 and 10.39 on the respective language translations to English. Multilingual modelling is also applied to translate these languages to English and we showed that vocabulary overlap contributes to the success of the multilingual model. Lastly, we applied back translation to augment the smallest dataset, which is the Luhya to English dataset. -
B 3 Francielle Vargas
Discourse-Aware Approaches for Multilingual Deception Detection[Abstract]
There are reliable cues for detecting deception and the belief that liars give off cues that may indicate their deception is near-universal. Furthermore, a fairly straightforward element to mitigate risks of deceptive activities is to identify deceptive intentions. A wide variety of models have been proposed in the literature to automatically identify statements that are intentionally misstated (or manipulated), and the vast majority of these models rely on linguistic features, such as n-grams, language complexity, part-of-speech tags, and syntactic, semantic, and psycholinguistics. In this Ph.D. ongoing, we propose to assess discourse-aware approaches to characterizing and predicting deceptive intentions. Our major contribution is to understand and explicitly measure discourse structure linguistic properties of deceptive versus real news sources while mitigating the topical bias. We aim to further analyze theoretically and compare empirically multilingual patterns in deceptive and truthful news. Accordingly, we intend to propose a discourse-aware model, in which deceptive discourse structure is a first-class citizen both in terms of natural language understanding. The current progress in this thesis, including (i) the construction of the first multilingual deceptive corpus, which was annotated by specialists according to the Rhetorical Structure Theory framework, and (ii) the introduction of two new proposed rhetorical relations: INTERJECTION and IMPERATIVE, which we assume to be relevant for the fake news detection task, (iii) assessing of contextualized embeddings performance for multilingual deception detection. -
B 4 Max Müller-Eberstein
Genre as Weak Supervision for Cross-lingual Dependency Parsing[Abstract]
Dataset genre labels are already frequently available, yet remain largely unexplored in cross-lingual setups. We harness this genre metadata as a weak supervision signal for targeted data selection in zero-shot dependency parsing. Specifically, we project treebank-level genre information to the finer-grained sentence level, with the goal to amplify information implicitly stored in unsupervised contextualized representations. We demonstrate that genre is recoverable from multilingual contextual embeddings and that it provides an effective signal for training data selection in cross-lingual, zero-shot scenarios. For 12 low-resource language treebanks, six of which are test-only, our genre-specific methods significantly outperform competitive baselines as well as recent embedding-based methods for data selection. Moreover, genre-based data selection provides new state-of-the-art results for three of these target languages. -
B 5 Mohammad Yeghaneh Abkenar
Neural Argumentation Mining on Essays and Microtexts[Abstract]
Detecting the argument components Claim and Premise is a central task in argumentation mining. We work with two annotated corpora from the genre of short argumentative texts. We extend a BiLSTMCRF neural tagger to identify argumentative units and to classify their type (claim vs. premise). we adopt contextual word embeddings (Bert, RoBerta) and cast the problem as a sequence labeling task -
B 6 Ilia Stepin
Argumentative explanatory dialogue modelling for responsible and trustworthy artificial intelligence[Abstract]
Communicating explanation in a comprehensible manner is essential for establishing human-machine interaction when justifying a prediction of an AI-based classifier. This issue gains importance when multiple different instances of explanation are to be offered. For example, a less relevant (from the artificial agent’s point of view) piece of counterfactual explanation can be seen more trustworthy/reliable from the user’s point of view. To address the issue of miscommunication in explaining the agent’s output, we propose an explanatory dialogue protocol based on insights from argumentation theory. The protocol is claimed to (1) enable the end-user to construct a big picture of the underlying reasoning of the given classifier and (2) offer a transparent means of communicating personalised factual and counterfactual explanations. -
B 7 Lisa Bylinina
Polarity-sensitivity in multilingual language models[Abstract]
To what extent do multilingual language models generalize similar phenomena across languages? What are these generalizations driven by? We focus on polarity-sensitivity as an example of a typologically common linguistic phenomenon. We study the behaviour of ‘negative polarity items’ (NPIs; for example, English ‘any’) in different contexts in four languages (English, French, Russian and Turkish) in two pre-trained models – multilingual BERT (Devlin et al., 2018) and XLM-RoBERTa (Conneau et al., 2019). We evaluate the models’ recognition of polarity-sensitivity and its cross-lingual generality. Further, using the artificial language learning paradigm, we look for the connection between semantic profiles of words and their ability to license NPIs. We only find evidence for such connection for sentential negation and not for other items we study. We conclude that generality of polarity-sensitivity in multilingual models is partial, and the relation between meaning and NPI hosting can only be seen for some licensers. Joint work with Alexey Tikhonov (Yandex) -
B 8 Elisa Bassignana
A Survey of Relation Extraction Datasets and an In-depth Study of the Scientific Domain[Abstract]
Over the last five years, research on Relation Extraction (RE) witnessed extensive progress. In this paper, we provide a comprehensive survey of RE datasets, with an additional focus on the task definition. We find unclarity in setups, which contributes to the difficulty of reliable comparison for RE (Taillé et al., 2020) and overestimation of generalization performance more generally in NLP (Gorman and Bedrick, 2019; Søgaard et al., 2021). We argue that cross-dataset (and whenever possible, cross-domain) evaluation is one way to address this issue. To exemplify this, we present an empirical study in scientific RE. We study two datasets, finding large overlaps, but also substantial discrepancies in annotations. We further propose new sub-domain splits. Our empirical analysis shows that annotation discrepancies strongly impact Relation Classification performance, explaining large drops in cross-dataset evaluations. Variation within sub-domains exists but impacts Relation Classification only to limited degrees. Overall, our study calls for more rigour in reporting setups in RE and evaluation across multiple test sets. -
B 10 Arash Ashrafzadeh
Incremental Processing in Dialogue Systems[Abstract]
Human process dialogue incrementally, on a token by token basis (e.g. Howes (2012)); and many works focus on incremental dialogue system architectures (Schlangen and Skantze, 2009; Baumann and Schlangen, 2012; Kennington et al., 2014) known to be more natural (Skantze and Hjalmarsson, 2010). For instance, Purver et al. (2011) present a domain-general approach, using Dynamic Syntax (Kempson et al., 2001) for incremental semantic processing. On the one hand, neural end-to-end dialogue system architectures (Vinyals and Le (2015); Lowe et al. (2017); Wolf et al. (2019) a.o.) are not incremental and do not generalise well (Eshghi et al., 2017). On the other hand, grammar-based approaches are difficult to implement, and remain brittle due to low coverage. Therefore, my research focuses on developing models for (1) Incremental, neural, semantic processing for dialogue (see Madureira and Schlangen (2020); Rohanian and Hough (2020)); and (2) wide-coverage incremental grammar learning, following Eshghi et al. (2013). -
B 11 Ekaterina Svikhnushina
Key Qualities of Conversational Chatbots – the PEACE Model[Abstract]
Open-domain chatbots engage in natural conversations with users to socialize and establish bonds. However, developing an effective chatbot is challenging. It is unclear what qualities of such chatbots most correspond to users’ expectations. Even though existing work has considered a wide range of aspects, their consistency and validity have not been tested. We describe a large-scale survey using a consolidated model to elicit users’ preferences, expectations, and concerns. We apply structural equation modeling to further validate the results. The outcome supports the consistency, validity, and reliability of the model, which we call PEACE (Politeness, Entertainment, Attentive Curiosity, and Empathy). PEACE, therefore, defines the key determinants most predictive of user acceptance and suggests implications for the development of compelling open-domain chatbots. -
B 12 Simran Khanuja
Evaluating Inclusivity, Equity, and Accessibility of NLP Technology: A Case Study for Indian Languages[Abstract]
In order for NLP technology to be widely applicable and useful, it needs to be inclusive of users across the world’s languages, equitable, i.e., not unduly biased towards any particular language, and accessible to users, particularly in low-resource settings where compute constraints are common. To address this, we propose an evaluation paradigm that assesses NLP technologies across all three dimensions, hence quantifying the diversity of users they can serve. While inclusion and accessibility have received attention in recent literature, equity is currently unexplored. We propose to address this gap using the Gini coefficient, a well-established metric used for estimating societal wealth inequality. Using our paradigm, we highlight the distressed state of diversity of current technologies for Indian (IN) languages. Our focus on IN is motivated by their linguistic diversity and their large, varied speaker population. To improve upon these metrics, we demonstrate the importance of region-specific choices in model building and dataset creation and also propose a novel approach to optimal resource allocation during fine-tuning. Finally, we discuss steps that must be taken to mitigate these biases and call upon the community to incorporate our evaluation paradigm when building linguistically diverse technologies. -
B 13 Rakia Saidi
Design of an automatic disambiguation system using neural networks[Abstract]
Deep neural networks have demonstrated unprecedented capacities in recent years, and are revolutionizing the field of AI. In the field of NLP, recent progress has made it possible to achieve performance that is often superior to that obtained by conventional methods, namely methods based on dictionaries and knowledge. The aim is to see the potential for progress of such a technology for the task of syntactic disambiguation (Part Of Speech POS tagging) and semantic disambiguation (Word Sense Disambiguation WSD) in the Arabic language. – The WSD is the process of identifying the sense of a word in context. – The POS tagging consists in assigning a lexical category also known as a part of speech to each word in a sentence. -
B 14 Michele Cafagna
Investigating the capabilities of V&L models to align scene descriptions to images[Abstract]
Images can be described in terms of the objects they contain, or in terms of the types of scene or place that they instantiate. We address to what extent pretrained Vision and Language models can learn to align descriptions of both types with images. We find that (i) V\&L models are susceptible to stylistic biases acquired during pretraining; (ii) only one of them performs consistently well on both object- and scene-level descriptions. A follow-up ablation study shows that it uses object-level information in the visual modality to align with scene-level textual descriptions. -
B 15 Evangelia Gogoulou
Cross-lingual transfer of monolingual models[Abstract]
Recent studies in zero-shot cross-lingual learning using multilingual models have falsified the previous hypothesis that shared vocabulary and joint pre-training are the keys to crosslingual generalization. Inspired by this advancement, we introduce a cross-lingual transfer method for monolingual models based on domain adaptation. We study the effects of such transfer from four different languages to English. Our experimental results on GLUE show that the transferred models outperform an English model trained from scratch independently of the source language. After probing the model representations, representations, we find that model knowledge from the source language is transferred to the target language and improves the model performance on syntactic and semantic probing in English. -
B 16 Khalid Ahmed
Neural Machine Translation Between Low-resource Languages[Abstract]
Machine Translation (MT) between low-resource languages is challenging because it is hard to find enough parallel data between two low-resource languages for training a direct translation model. Pivot-based MT approaches have proven their effectiveness when the parallel data between source and target languages are absent. Other works did not consider making any changes in the pivot language sentences before training. Based on the fact that translating between structurally similar languages is easier for NMT models, we are aiming to modify pivot sentences before training to make them structurally similar to the source language, target language, or something in between. We will use two approaches to do this modification: regeneration of pivot sentences using Sequence-Level Knowledge Distillation (KD) and reordering. -
B 17 Ryan Teehan
Cut the CARP: Fishing for zero-shot story evaluation[Abstract]
Recent advances in large-scale language models (Raffel et al., 2019; Brown et al., 2020) have brought significant qualitative and quantitative improvements in machine-driven text generation. Despite this, generation and evaluation of machine-generated narrative text remains a challenging problem. Objective evaluation of computationally-generated stories may be prohibitively expensive, require meticulously annotated datasets, or may not adequately measure the logical coherence of a generated story’s narratological structure. Informed by recent advances in contrastive learning (Radford et al., 2021), we present Contrastive Authoring and Reviewing Pairing (CARP): a scalable, efficient method for performing qualitatively superior, zero-shot evaluation of stories. We show a strong correlation between human evaluation of stories and those of CARP. Model outputs more significantly correlate with corresponding human input than those language-model based methods which utilize finetuning or prompt engineering approaches. We also present and analyze the Story-Critique Dataset, a new corpora composed of 1.3 million aligned story-critique pairs derived from over 80,000 stories. We expect this corpus to be of interest to NLP researchers. -
B 18 Joonas Kalda
Collar-Aware Training for Speaker Change Detection in Broadcast Speech[Abstract]
The main challenges of speaker change detection are the vagueness of annotated change points caused by the silences between speaker turns and imbalanced data due to the majority of frames not including a speaker change. Conventional training methods tackle these by artificially increasing the proportion of positive labels in the training data. Instead, the proposed method uses an objective function that encourages the model to predict a single positive label within a specified collar. This is done by summing up the standard binary sequence labelling objective over all paths that have exactly one positive label inside the collar. Experiments on English and Estonian datasets show large improvements over the conventional training method. Additionally, the model outputs have peaks concentrated to a single frame, removing the need for post-processing to find the exact predicted change point which is particularly useful for online applications
Social Sessions
Social 1
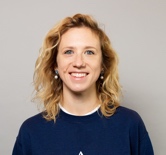
Sasha Luccioni – Green NLP
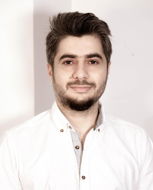
Raheel Qader – NLP & Finance
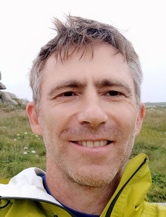
Shachar Mirkin – Being a Remote Scientist
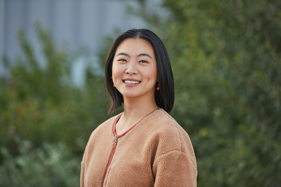
Angela Fan – Multilingual NLP
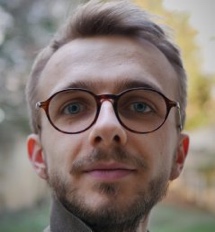
Titouan Parcollet – Open Science and Speech Technologies
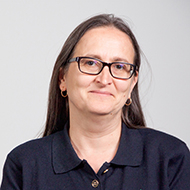
Gabriela Csurka Khedari – Image and Text
Social 2
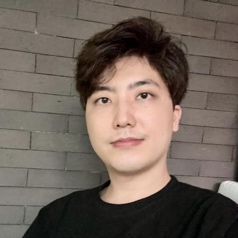
Kang Min – HyperClova
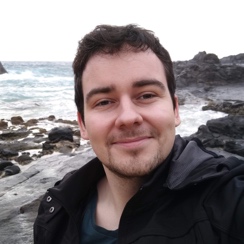
Sebastian Ruder– NLP for under-represented languages
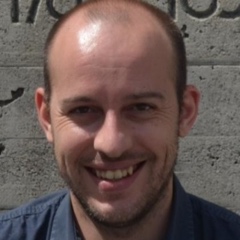
Fabien Ringeval – How to prepare your academic career in France
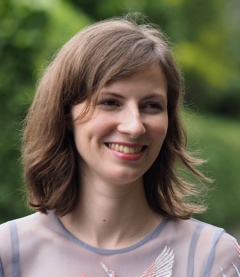
Rachel Bawden – Data resources in NLP: how to construct them and how to evaluate resource papers.
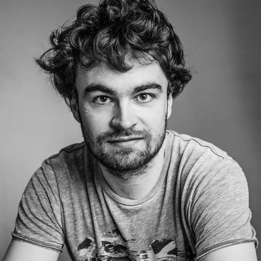
William Havard – Managing an interdisciplinary PhD
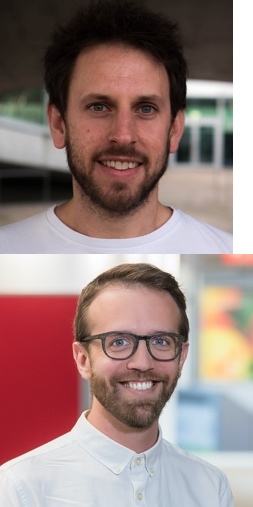
Leandro Von Werra & Lewis Tunstall – Accelerating your research with the Hugging Face ecosystem